Sustainable AI: The Eco-Friendly Impact of Generative AI Chip Sales
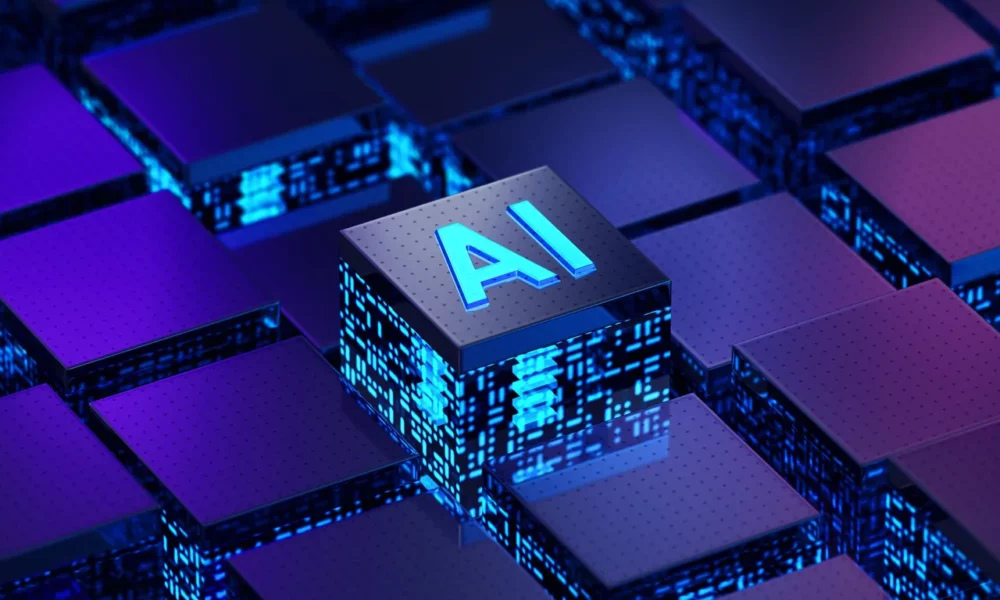
GenAI: A Creative Powerhouse in the Tech World
Generative AI (GenAI) has emerged as a powerful force in the tech landscape, capturing the imagination of consumers and businesses alike. Pioneering models like ChatGPT and Bard have brought AI-powered text generation to the forefront, blurring the lines between human and machine creativity. This newfound ability to create novel content from scratch marks a significant departure from traditional AI, which relies heavily on pre-programmed rules.
GenAI excels at learning from existing data and producing entirely new outputs, often with surprising originality. This translates to significant time and cost savings across various content creation domains – text, code, audio, images, and even video. Experts believe the potential applications of GenAI are vast, spanning customer service, marketing, software engineering, product development, and countless other functions.
While the potential scope of ChatGPT and other GenAI models is significant, it is crucial to consider the technology’s environmental sustainability to ensure responsible development and use.
Can We Innovate Sustainably?
Experts caution users about the exposure of valuable data, such as private customer information and company trade secrets, to potential hackers when utilizing GenAI tools. Additionally, the environmental ramifications of these technologies are increasingly apparent. As societal pressure to monitor carbon emissions and address environmental concerns mounts, companies must confront the significant resource requirements and carbon footprint associated with GenAI.
Generative AI relies on powerful servers, raising concerns about increased energy consumption and its impact on the environment. For instance, according to a study published in Joule, AI constituted 10 to 15 percent of Google’s electricity usage in 2021, a figure expected to rise due to the company’s expanding AI initiatives. Data centers hosting generative AI models consume substantial energy and water, primarily for cooling and non-renewable electricity generation, contributing to global greenhouse gas emissions.
Most prominent GenAI models are developed by large cloud providers, utilizing thousands of servers with significant carbon footprints, particularly due to their reliance on graphics processing unit (GPU) chips. These GPUs consume 10–15 times more energy than traditional CPUs, resulting in considerable carbon emissions during model training. Moreover, the rapid hardware replacement cycle in GenAI exacerbates e-waste concerns.
Despite growing interest in sustainability, executives primarily prioritize understanding the technology’s impact on their industry and operations, aiming to differentiate products and enhance efficiency while upholding security and privacy standards. Trustworthiness is a key consideration for business leaders when evaluating GenAI projects.
While generative AI companies often withhold detailed information about energy consumption and specialized chip usage, projections made using data from chipmaker Nvidia suggest that AI servers could consume between 85 and 134 terawatt hours annually by 2027. As sustainability gains traction, attention to GenAI’s environmental impact is likely to increase.
How AI Chips are Going Green
As AI workloads become more complex, concerns rise about the environmental impact of data centers that power them. To combat this, chipmakers are developing a new generation of AI accelerator chips with powerful processors specifically designed for AI tasks, but with a focus on energy efficiency. This trend is driving significant growth in the AI accelerator chip market.
Here are some recent advancements:
Brain-inspired chips
IBM’s NorthPole chip boasts over 20x the speed and 25x the energy efficiency of existing AI chips. This innovation merges brain-inspired computing with traditional methods, minimizing energy waste during data transfer. NorthPole excels in tasks like image analysis and speech recognition, making it ideal for applications in autonomous vehicles and digital assistants.
Specialized AI processors
Kneron, an AI chip startup, recently unveiled the KL730 chip. This chip, designed for large-scale AI applications, challenges the dominance of GPUs (Graphical Processing Units) in AI. Kneron claims that KL730 delivers significant cost savings (up to 75%) compared to GPUs, while also being 150-200% more energy-efficient than competitor products. The KL730 is expected to be particularly impactful in autonomous vehicles, for features like full-frame detection in driver-assistance systems.
Beyond chip architecture, advancements in materials and manufacturing are also contributing to energy efficiency. Some chipmakers are exploring new materials like gallium nitride (GaN) to reduce power consumption without compromising the performance. Others are investigating 3D packaging technology, which shortens the distance electrical signals travel within a chip, further reducing energy use.
The focus on energy efficiency in AI chip development is crucial for a more sustainable future of AI. This trend is fostering fierce competition among chipmakers, leading to a constant stream of innovations and advancements. The race for the most energy-efficient AI accelerator chip is ultimately propelling the entire industry forward, making AI not only more powerful but also more environmentally responsible.
Some other advancements include:
- Intel Loihi Chip: This neuromorphic chip is designed for applications like robotics and autonomous vehicles, focusing on lower power consumption.
- Google TPU v4 Chip: This custom-designed chip is used by Google for training large language models while keeping energy efficiency in mind.
- NXP RT6000 Chip: This chip prioritizes low power consumption while delivering high performance for edge AI applications.
Sustainability Through Efficiency
While GenAI can have environmental drawbacks due to its reliance on data centers, the chips that power these models are undergoing transformation with positive environmental implications. The key lies in energy efficiency.
AI for a Greener Future
Even though GenAI has been branded as environmentally damaging, it also offers solutions that can foster sustainability in various sectors. Here are some examples:
Smarter Farming: AI analyses agricultural land, optimizing water usage, reducing waste, and increasing crop yields. Google’s India project, using AI and satellite imagery, empowers farmers to prepare for and mitigate droughts.
Precision Agriculture: Vietnamese startup MimosaTEK offers AI-powered tools that monitor soil conditions and other crucial parameters. This empowers farmers to practice precision agriculture, which optimizes resource use and boosts productivity.
Waste Management: AI plays a vital role in identifying opportunities for recycling and waste reduction. Companies like Waste Labs utilize data to streamline waste collection within cities, lowering costs and promoting sustainable waste management practices.
Traffic Management: Congestion, a key source of urban air pollution, can be mitigated with electric vehicles and AI. NoTraffic, an Israeli firm, analyzes traffic data to optimize traffic lights, enhancing fuel efficiency and traffic flow.
These examples showcase how AI, when combined with energy-efficient chip technology, can contribute to a more sustainable future.
While GenAI offers a pathway to a greener future, addressing its environmental impact remains crucial. A roadmap outlines key steps for a company to develop and utilize artificial intelligence (AI) in a sustainable manner. It emphasizes on minimizing environmental impact while maximizing the benefits of AI technology.
Phase 1: Planning and Assessment
- Define Sustainability Goals: Establish clear metrics for measuring the environmental impact of your AI development and usage. This could include energy consumption, carbon footprint, and responsible data management
- AI Use Case Evaluation: Analyze potential AI applications through a sustainability lens. Prioritize projects that can optimize resource usage, reduce waste, or contribute to environmental solutions
- Team Building: Assemble a team with expertise in AI, sustainability, and responsible data practices. Foster collaboration to ensure ethical and environmentally conscious development
- Vendor Selection: When choosing AI hardware and software providers, consider their commitment to sustainability practices. Look for energy-efficient hardware and cloud services with renewable energy sources
Phase 2: Development and Implementation
- Data Optimization: Implement techniques for data efficiency. This includes data cleaning, compression, and using smaller datasets while maintaining AI accuracy
- Hardware Selection: Prioritize energy-efficient AI chips and hardware designed for low power consumption. Research options like neuromorphic computing for potentially lower energy needs
- Model Optimization: Design and train AI models with a focus on efficiency. Techniques like model pruning and quantization can reduce the computational footprint without sacrificing performance
- Monitoring and Optimization: Continuously monitor the environmental impact of your AI systems. Identify opportunities for enhancement and deploy continuous optimization tactics
Phase 3: Continuous Improvement
- Knowledge Sharing: Promote best practices for sustainable AI within your company and collaborate with industry partners to share knowledge
- Research and Development: Invest in research and development of AI algorithms and hardware that are specifically designed for sustainability
- Advocacy: Advocate for policy changes and industry standards that promote the development and application of sustainable AI technologies
- Reporting and Transparency: Regularly report on your progress towards achieving sustainability goals and promote transparency within your AI development process
Additional Consideration
- Lifecycle Management: Develop a plan for the entire lifecycle of your AI systems, including responsible disposal or repurposing of hardware at the end of its useful life
- Explainable AI: Implement explainable AI (XAI) techniques to understand and monitor the decision-making process of your AI systems. This promotes responsible use and reduces bias
- Training Data Bias: Be mindful of potential bias within the training data used for your AI models. Implement strategies to mitigate bias and ensure fair and ethical outcomes
By following these steps, companies can embark on a journey towards creating and using AI that benefits both society and the environment. Remember, sustainable AI is an ongoing process that requires continuous learning and adaptation.
GenAI: A Double-Edged Sword for Sustainability
AI offers immense potential, but its environmental impact can’t be ignored. Here’s a closer look at this technology’s role in sustainability:
The Downside: Environmental Footprint
Training GenAI models consumes significant amounts of energy. This raises concerns about its environmental impact, especially regarding:
- Data Center Efficiency: The energy use and greenhouse gas emissions of data centers housing these models need to be addressed. Solutions include using renewable energy sources, energy-efficient technologies, and carbon capture methods
- Hardware Sustainability: The materials used in hardware devices and their recyclability need to be improved. Exploring alternative materials, modular design, and circular economy models can minimize environmental impact
The Upside: Sustainability Champion
GenAI can be harnessed to promote environmental responsibility:
- ESG Initiatives: GenAI can support Environmental, Social, and Governance (ESG) efforts by suggesting innovative solutions for environmental challenges. Examples include calculating carbon footprint, designing low-impact processes, and automating ESG reporting.
- Mid-Market Benefits: Mid-sized organizations, often lacking resources for extensive sustainability efforts, can leverage generative AI to address environmental concerns effectively.
The Future of Greener GenAI
The environmental impact of generative AI is expected to lessen as the technology evolves. Advancements in areas like:
- Low-Power Electronics: Developing low-power electronics specifically designed for AI applications.
- System Efficiency: Creating systems optimized for energy efficiency to reduce overall power consumption.
- Resource Utilization: Improving the utilization of compute resources to minimize wasted energy.
- Efficient Code: Writing code specifically designed for efficiency within AI applications.
- Energy-Efficient Chips: Researching and developing energy-efficient AI chips, such as neuromorphic chips, analog chips, and in-memory computing solutions.
By prioritizing these areas, businesses and tech stakeholders can play a vital role in shaping a greener future for generative AI. This approach ensures that the benefits of this technology can be enjoyed without compromising environmental well-being.
Conclusion
GenAI presents a unique opportunity to address environmental issues while offering its own set of challenges. By prioritizing sustainability and adopting these advancements, we can ensure GenAI becomes a force for good, curbing its environmental impact while maximizing its positive contributions.
Embrace digital transformation powered by Netscribes’ AI solutions with sustainable business strategies .
Read More From Techbullion And Businesnewswire.com